SearchAI Private LLM
High-Performance LLM Inference with Purely CPUs
Enable AI Assistants easily and securely on your organization’s private data.
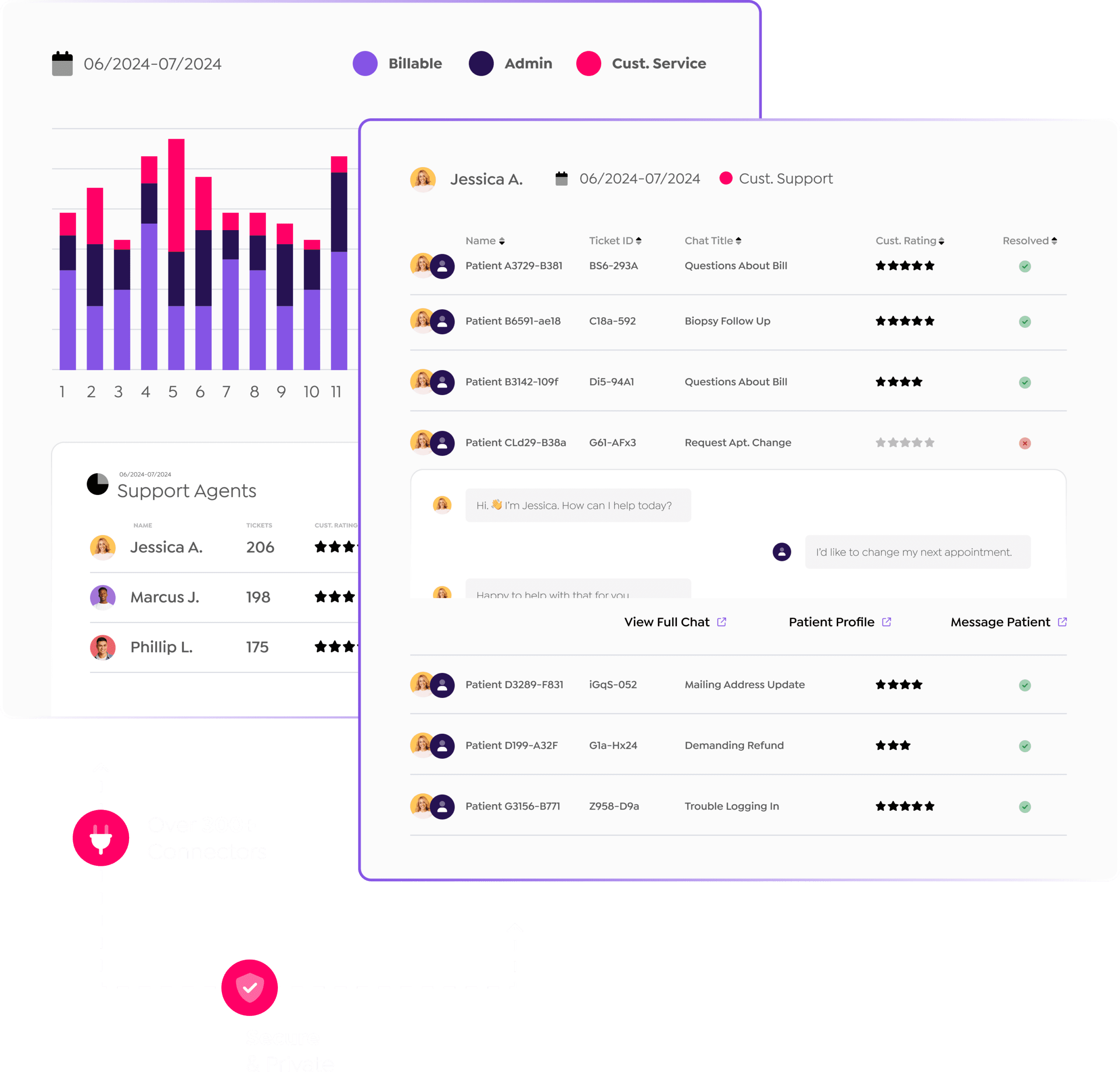
Fixed Cost
Significantly reduce costs and complexity; our solutions easily integrate with existing hardware and virtual servers.
Highly Scalable
Deploy AI models that are scalable across your organization and unlock the full potential of your data.
Secure & Private
Keep your model, your inference requests and your data sets within the security domain of your organization.
Optimized for RAG
Set up AI assistants and conversational chatbots using your own data directly on the SearchAI platform.
What is RAG?
RAG stands for Retrieval-Augmented Generation. It is an approach that combines the capabilities of large language models (LLMs) with information retrieval from external data sources. Here’s how it works:
This allows RAG models to provide more factual and up-to-date responses by leveraging external knowledge sources, rather than being limited to what’s contained in the language model’s training data.
Not sure what's best?
Schedule a private consultation to see how SearchAI will make a difference across your enterprise.
Setting Up RAG ChatBots .
SearchAI provides a single platform for deploying RAG chatbots on your data, making it easy to leverage the power of RAG without compromising data privacy or security. Set up in a few simple steps.
Data Ingestion
Import your proprietary data sources or document corpora into the SearchAI platform. This could include manuals, legal documents, knowledge bases, etc.
Index Creation
Build efficient search indexes over your data to enable fast retrieval.
Model Integration
Connect and use SearchAI Private LLM.
ChatBot Interface Setup
Setup your chatbot for the private data using SearchAI.
Query Handling
When a user query comes in, SearchAI retrieves relevant information and passes it to your LLM to generate a final response.
Testing & Refinement
Evaluate your chatbot’s performance, fine-tune prompts and make adjustments to improve accuracy.
Deployment
Once finalized, deploy your private RAG chatbot on your own hardware or SearchAI’s secure cloud infrastructure.
The Benefits of RAG
There are several key benefits to using a RAG approach:
Improved Accuracy & Relevance
Access to Up-to-Date Information
Domain Knowledge Expansion
No Hallucination
Enhanced Reasoning Capabilities
Improved Accuracy & Relevance
By retrieving information from pre-defined authoritative sources, RAG can provide more factual and reliable responses than LLMs alone.
Access to Up-to-Date Information
The retrieval component allows RAG systems to utilize the latest information from multiple data sources using the most appropriate retrieval algorithm for structured and unstructured data.
Domain Knowledge Expansion
RAG can leverage specialized document corpora to provide personalized responses for specific domains, such as legal, medical, engineering or technical fields.
No Hallucination
Retrieving evidence from actual sources eliminates the chances of language models generating implausible or hallucinatory outputs.
Enhanced Reasoning Capabilities
Combining retrieval with large language models enables more complex multi-step reasoning and question-answering abilities.
Use Cases for SearchAI Private LLM
The benefits of AI assistants range from increased customer experience and faster service ticket responses to higher employee output and increased organizational knowledge. How will you leverage SearchAI to grow your organization?
See For Yourself
Discover the strategic benefits of RAG — get started with a private, customized demo with your own data.
SearchAI Private LLM
High-Performance LLM Inference with Purely CPUs
Enable AI Assistants easily and securely on your organization’s private data.
Fixed Cost
Significantly reduce costs and complexity; our solutions easily integrate with existing hardware and virtual servers.
Highly Scalable
Deploy AI models that are scalable across your organization and unlock the full potential of your data.
Secure & Private
Keep your model, your inference requests and your data sets within the security domain of your organization.
Optimized for RAG
Set up AI assistants and conversational chatbots using your own data directly on the SearchAI platform.
What is RAG?
RAG stands for Retrieval-Augmented Generation. It is an approach that combines the capabilities of large language models (LLMs) with information retrieval from external data sources. Here’s how it works:
01
Asking Questions
A user starts a conversation with the chatbot through a question. The user question is converted into a semantic retrieval query.
02
Fetching Answers
The retrieval component searches through a corpus of documents or data sources to find the most relevant information.
03
Creating Context
The retrieved information, along with the user question, is passed to a large language model (LLM) for processing.
04
Delivering Results
The language model generates a final answer by synthesizing and reasoning over the retrieved information.
This allows RAG models to provide more factual and up-to-date responses by leveraging external knowledge sources, rather than being limited to what’s contained in the language model’s training data.
Setting up RAG ChatBots efficiently and securely.
SearchAI provides a single platform for deploying RAG chatbots on your data, making it easy to leverage the power of RAG without compromising data privacy or security. Set up in a few simple steps.
Data Ingestion
Import your proprietary data sources or document corpora into the SearchAI platform. This could include manuals, legal documents, knowledge bases, etc.
Index Creation
Build efficient search indexes over your data to enable fast retrieval.
Model Integration
Connect and use SearchAI Private LLM.
ChatBot Interface Setup
Setup your chatbot for the private data using SearchAI.
Query Handling
When a user query comes in, SearchAI retrieves relevant information and passes it to your LLM to generate a final response.
Testing & Refinement
Evaluate your chatbot’s performance, fine-tune prompts and make adjustments to improve accuracy.
Deployment
Once finalized, deploy your private RAG chatbot on your own hardware or SearchAI’s secure cloud infrastructure.
The Benefits of RAG
There are several key benefits to using a RAG approach:
Improved Accuracy & Relevance
Access to Up-to-Date Information
Domain Knowledge Expansion
No Hallucination
Enhanced Reasoning Capabilities
Improved Accuracy & Relevance
By retrieving information from pre-defined authoritative sources, RAG can provide more factual and reliable responses than LLMs alone.
Access to Up-to-Date Information
The retrieval component allows RAG systems to utilize the latest information from multiple data sources using the most appropriate retrieval algorithm for structured and unstructured data.
Domain Knowledge Expansion
RAG can leverage specialized document corpora to provide personalized responses for specific domains, such as legal, medical, engineering or technical fields.
No Hallucination
Retrieving evidence from actual sources eliminates the chances of language models generating implausible or hallucinatory outputs.
Enhanced Reasoning Capabilities
Combining retrieval with large language models enables more complex multi-step reasoning and question-answering abilities.
Use Cases for SearchAI Private LLM
The benefits of AI assistants range from increased customer experience and faster service ticket responses to higher employee output and increased organizational knowledge. How will you leverage SearchAI to grow your organization?
Government
Maintaining compliance clarity.
A government agency launched a chatbot for tax professionals to ask questions on complex laws, rulings and decisions.
Manufacturing
Aligning organizational knowledge.
A manufacturing facility needs to find information from product manuals in an expedited manner.
Finance
Expediting customer support responses.
A finance department can query information about purchase orders and invoices for efficient customer support.
See For Yourself
Discover the strategic benefits of RAG — get started with a private, customized demo with your own data.